DeepSeek-R1: The Next Big Thing in AI—Or Just Hype?
1. The Launch and Initial Breakthroughs
On January 20, 2025, Chinese AI startup DeepSeek released its open-source reasoning model, DeepSeek-R1, It claims to perform as well as OpenAI’s O1 model. Just days later, on January 23, DeepSeek published a detailed report that highlighted several groundbreaking advancements.
One of the most eye-catching discoveries was DeepSeek-R1-Zero—a model trained entirely through “Reinforcement Learning (RL) without any initial dataset”. RL allows computers to learn by interacting with an environment and receiving feedback, much like how humans learn through trial and error. DeepSeek-R1 itself pushed the boundaries even further by combining initial training data with RL fine-tuning, making it nearly as powerful as OpenAI’s more advanced o1-1217 model. This underscores the growing importance of reinforcement learning in building highly capable AI systems—even in the absence of large, curated datasets.
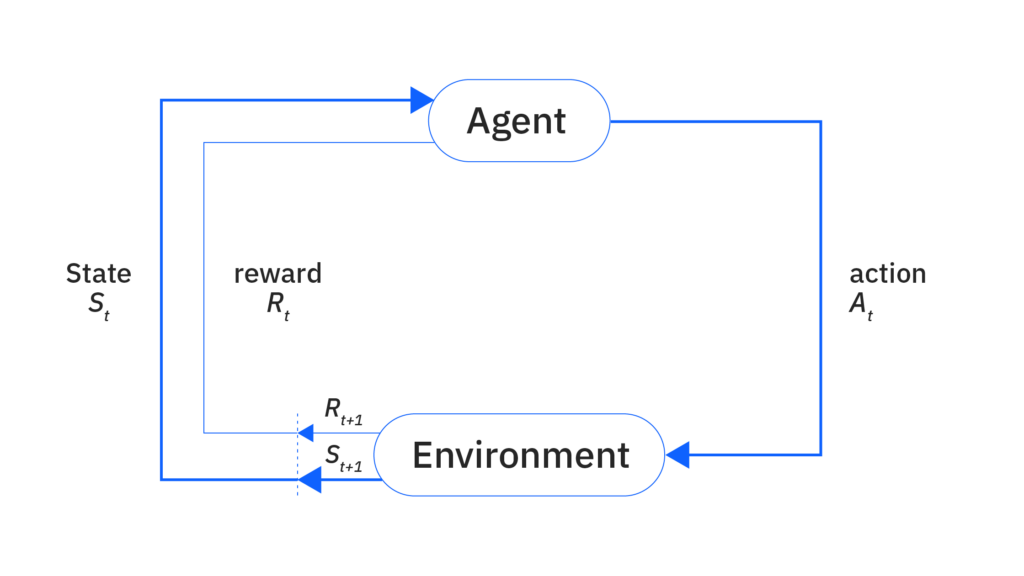
2. Extending Reasoning Through Distillation
In a bold experiment, DeepSeek set out to transfer R1’s robust reasoning capabilities to smaller models. Using DeepSeek-R1 as a teacher, the team generated 800,000 training examples to fine-tune more compact models. The results were striking: DeepSeek-R1-Distill-Qwen-1.5B outperformed GPT-4o and Claude-3.5-Sonnet in mathematical reasoning. Other distilled variants also surpassed existing instruction-tuned models. This success suggests that expert-level reasoning can be distilled into smaller, more efficient systems.
DeepSeek also shared its multi-stage optimization pipeline for refining the DeepSeek-R1 model. While DeepSeek-R1-Zero showed promise, it initially struggled with issues like language mixing and messy outputs. To address these challenges, DeepSeek employed the following stages in its optimization process:
- Cold Start Fine-Tuning: DeepSeek created a small, high-quality dataset with long Chain-of-Thought (CoT) examples and readable data to stabilize the model’s early development.
- Reasoning-Oriented Reinforcement Learning: In this stage, DeepSeek applied RL to enhance the model’s reasoning abilities, particularly in math and coding, and introduced a “language consistency reward” to reduce language mixing.
- Rejection Sampling and Supervised Fine-Tuning: Following the RL phase, DeepSeek generated a large dataset via rejection sampling and fine-tuned the model to improve its performance across various domains.
- Second RL Phase: The final stage involved another round of RL to refine the model’s alignment and overall performance.
Deep Seek’s systematic approach to guiding the model through these stages, rather than relying on a simple RL process, was crucial to its success.
3. Market Reactions & Industry Impact
By January 28, 2025, financial markets were already feeling the effects of DeepSeek’s AI advances. U.S. tech stocks temporarily dipped, shedding around $1 trillion in market value before showing signs of recovery. Notably, President Donald Trump weighed in, calling the DeepSeek AI chatbot a “wake-up call” for Silicon Valley and emphasizing the intensifying global race to dominate AI technologies.
4. Beyond the Hype: Real-World Implications
While DeepSeek-R1 sparked excitement for its OpenAI-rivaling claims, hands-on testing by Viceroy Solutions and QureNote AI developers uncovered a more complex reality. Despite its technological promise, real-world integration revealed significant hurdles.
4.1. QureNote AI’s Integration Roadblocks
- QureNote AI’s senior developers faced challenges integrating DeepSeek-R1 into the automation workflow tools such as n8n, etc. The Base URL parameters didn’t connect properly, causing delays. After multiple attempts, they managed to establish the connection, but the process took significant time. However, the model’s API call was slower than expected, and responses were inaccurate for healthcare use, and real-time applications.
- In contrast, OpenAI’s GPT models integrated smoothly, highlighting a gap between DeepSeek-R1’s potential and its readiness for enterprise use. For healthcare AI and workflow automation, reliable connectivity is crucial for seamless functionality.
4.2. Viceroy Solutions’ Challenges
- Engineers at Viceroy Solutions faced similar issues while running DeepSeek’s models on Ollama, an open-source platform for local LLM operations. Integration challenges raised doubts about DeepSeek-R1’s readiness for real-world deployment.
- For organizations adopting AI solutions, these challenges go beyond technical issues—they become obstacles to innovation. If a model cannot be easily integrated into existing workflows, its research potential may not translate into real-world impact.
4.3 Developer Insights: Performance Testing of Different DeepSeek-R1 Models
- As AI models evolve, developers are exploring efficient ways to run them locally. Engineers at Viceroy Solutions and QureNote AI tested the DeepSeek-R1 70B model on Ollama, an AI inference framework designed for local deployment. This evaluation assessed its real-world feasibility by examining accuracy, processing speed, and resource consumption.
- Compared to the smaller 7B model, the 70B variant requires significantly more computing power. It demands 43 GB of storage, whereas the 7B model only needs 4.7 GB. While Ollama itself has a minimal footprint of 745 MB, the sheer size of the 70B model raises concerns about its practicality on consumer-grade hardware.
- The evaluation revealed a trade-off between efficiency and accuracy. Both the 7B and 70B models successfully analyzed folder structures and provided insights into code organization. However, when tested on complex queries requiring reasoning and deeper comprehension, both struggled to generate precise responses.
Performance Insights
- The 7B model showed reasonable CPU and RAM usage, making it a practical option for mid-range devices. In contrast, the 70B model required a massive 55 GB of RAM, making it unsuitable for standard hardware and better suited for high-end workstations or cloud-based AI deployments.
- Despite its higher computational demands, the 70B model did not deliver a proportional improvement in response quality, raising concerns about its practical advantages over the smaller variant specafically on code generation and analysis.
I have tested the DeepSeek web interface, it gives responses similar to OpenAI’s GPT-4o and works well for coding problems. While it provides meaningful answers on the web, it has trouble integrating with the systems & tools. The only problem I noticed was that it took longer to respond than GPT, likely due to high server traffic.
Sr. Developer, Viceroy Solutions Inc
5. Final Thought
DeepSeek-R1 has made significant progress in AI reasoning, especially with reinforcement learning and distillation techniques. However, its real-world usability is still uncertain. While the DeepSeek web app responds nearly as well as OpenAI’s models, it struggles with Deepseeks API’s integration into other platforms and has slower response times, likely due to our test server specification.
As AI advances, true innovation will depend on how easily models can be deployed, scaled, and used in enterprise settings. DeepSeek-R1 shows great promise, but until it integrates smoothly into the broader tech ecosystem, its long-term impact remains uncertain.